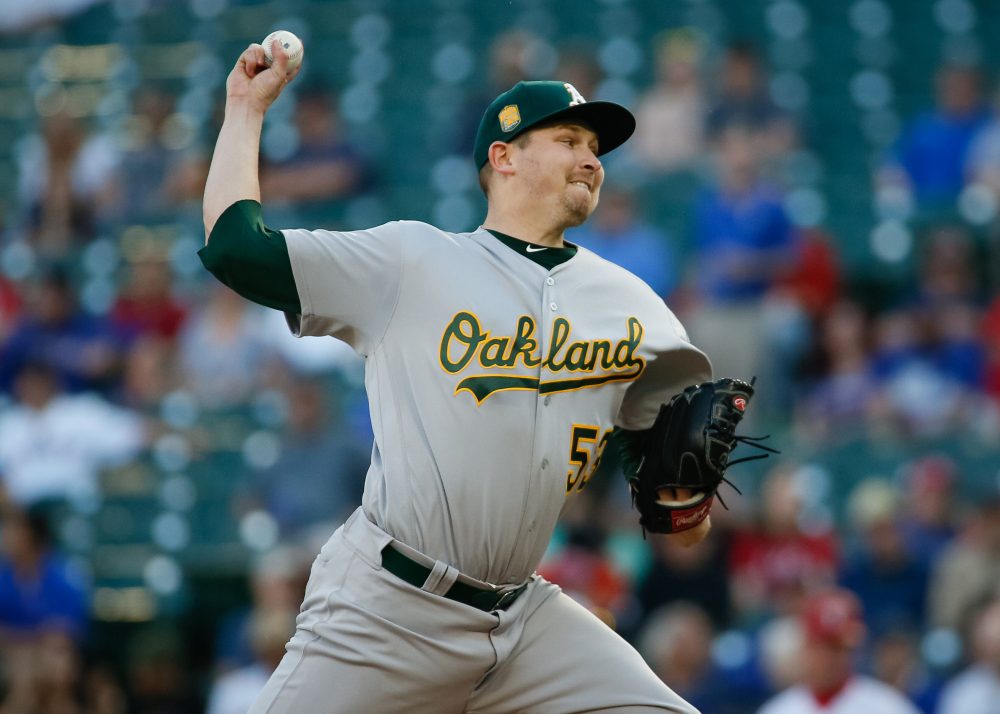
I’ve been playing fantasy baseball for such a long time that I can remember when FIP and BABIP were both a Really Big Deal.
It seems difficult to believe now but a few short years ago, fantasy analysts would simply utter a pitcher’s FIP and this was considered valid shorthand for player performance. BABIP didn’t possess quite the same degree of shorthand FIP did but it was still considered an ironclad metric that could tell you whether a player’s batting average was a true testament to his skill level or not. This was less than a decade ago.
In 2018, it is extremely rare to hear an analyst cite FIP as a marker for pitcher performance. BABIP is used occasionally but almost always with caveats surrounding its limited utility. In terms of understanding these specific metrics and their uses and limitations, we have come a long way.
This doesn’t mean fantasy players have solved for misunderstanding how to use data or what its practical applications are. In the endless search for an edge, nearly any new metric is immediately embraced, applied and then used as a cudgel to prove whatever point it is a fantasy analyst is attempting to make on any given day.
The latest attempt to try and solve for “how to win my fantasy league” is the new Statcast “X” metrics produced by MLB Advanced Media (BAM). Expected batting average (xBA) and expected weighted on-base average (xwOBA) are cited with increasing frequency to try and prove a player is trending positively or negatively and there will be a tangible change in his numbers going forward.
Last month, I wrote a column called Eureka Moments. To summarize, my point was that at the beginning of every baseball season, we waste a significant amount of time attempting to validate our drafts by leaning heavily on small sample size data to show the world that the players we drafted in March are good and the ones we avoided are bad. A colloquialism for this practice is “taking a victory lap”, which is something everyone loves to do on occasion but something fantasy analysts seemingly live for in April. There have been a lot of victory laps where Ozzie Albies is concerned and a lot of silence whenever Luis Castillo’s name comes up.
I digress. Whereas these “eureka moments” are attempts to prove a point by looking backward, “x” metrics are an attempt to prove a point by looking forward using batted ball data and exit velocity as part of the attempt. However, while the rationale surrounding this type of analysis is different, the point of it is virtually identical. See? I was right! Or at least I will be right in the future.
The problem with xBA and xwOBA is that while they are indeed useful metrics, they are far more useful as descriptive metrics than they are as predictive ones. My colleague Jonathan Judge does the heavy lifting in his article, but it is worth culling a couple of key points from there to illustrate this point.
Table 1: Correlation of xwOBA to Other Metrics
Statistic |
Correlation to Y+1 wOBA |
Margin of Error (plus/minus) |
xwOBA |
.35 |
.047 |
wOBA |
.32 |
.047 |
FIP |
.36 |
.045 |
DRA |
.36 |
.046 |
I highly recommend diving into Judge’s excellent piece to hear his far more informed words on the subject, but the bottom line is that expected wOBA and wOBA are almost equally accurate, within the margin of error. Put simply, if you are a fantasy player trying to figure out how a pitcher is going to perform for the rest of 2018, you are just as likely to find success using xwOBA as you are wOBA. Heck, you could party like it is 2010 and use FIP and come up with extremely similar results. That’s right, the metric I derided above, the one that uses home runs, strikeouts and walks and omits everything else is nearly as useful as the contemporary metric that attempts to use launch angle and exit velocity to predict future performance.
xBA and xwOBA are good at describing what already happened. But this isn’t as important as you might think.
Table 2: Descriptive Reliability of Metrics
Statistic |
Correlation to same-year wOBA |
Margin of Error (plus/minus) |
wOBA |
1.0 |
.000 |
xwOBA |
.83 |
.014 |
FIP |
.81 |
.014 |
DRA |
.74 |
.017 |
While xwOBA correlates more closely to xOBA than it does to FIP and DRA, it isn’t nearly as good as wOBA. xwOBA is pretty good at describing pitcher performance, but as Judge points out, you don’t need a tool that is “pretty good” at describing performance when you already have a tool (xOBA) that serves this purpose. FIP – the rudimentary metric the analytic community rightfully cast aside a few years ago because it uses home runs, strikeouts and walks and ignores everything else – is almost as useful as xwOBA, even from a descriptive standpoint.
From a data mining perspective, Judge’s study was exhaustive, looking at “complete batted ball summaries for pitchers from Baseball Savant for the 2015, 2016 and 2017 seasons.” But what if, as a fantasy player, I decided to look solely at the extremes? What if instead of looking at every pitcher, I looked at the pitchers who had the greatest difference between wOBA and wxOBA to see if there is a trend I could use in my fantasy leagues?
Table 3: 2017 wOBA and xwOBA outliers and 2018 results*
Pitcher |
2017 wOBA |
2017 xwOBA |
2018 wOBA |
“Winner” |
Trevor Cahill |
.364 |
.319 |
.266 |
wxOBA |
Clayton Richard |
.357 |
.325 |
.338 |
wxOBA |
Bartolo Colon |
.381 |
.353 |
.261 |
wxOBA |
Jameson Taillon |
.337 |
.310 |
.297 |
wxOBA |
Marco Estrada |
.333 |
.306 |
.364 |
wOBA |
Kyle Freeland |
.340 |
.320 |
.282 |
wxOBA |
Nick Pivetta |
.358 |
.340 |
.287 |
wxOBA |
Matt Boyd |
.350 |
.333 |
.260 |
wxOBA |
German Marquez |
.341 |
.326 |
.351 |
wOBA |
Jacob deGrom |
.291 |
.276 |
.237 |
wxOBA |
*minimum 1,500 total pitches in 2017; minimum 400 total pitches in 2018
Table 3 shows us the 10 pitchers with the largest differential between their 2017 wOBA and their 2017 xwOBA and shows how they are doing thus far in 2018. While I implicitly trust Judge’s research, now I am starting to wonder if there is something to the idea that xwOBA does have some practical applications. Eight of the 10 pitchers on Table 3 have a wOBA in 2018 that is closer to their 2017 xwOBA than their 2017 wOBA. Maybe there is something after all to the idea that xwOBA is predictive. Eureka!
Am I doing something wrong here and missing some obvious point? This can’t be the conclusion, can it? There must be a key piece of information I’m forgetting, something that I’m omitting. I’ll get to it eventua—
Oh, duh.
Table 4: 2016 wOBA and xwOBA outliers and 2017 results**
Pitcher |
2016 wOBA |
2016 xwOBA |
2017 wOBA |
“Winner” |
Michael Pineda |
.335 |
.304 |
.325 |
wOBA |
Anibal Sanchez |
.353 |
.325 |
.380 |
wOBA |
Matt Harvey |
.341 |
.316 |
.378 |
wOBA |
Luis Perdomo |
.360 |
.334 |
.339 |
wxOBA |
James Paxton |
.306 |
.282 |
.263 |
wxOBA |
CC Sabathia |
.312 |
.288 |
.309 |
wOBA |
Matt Cain |
.367 |
.343 |
.358 |
wOBA |
Jaime Garcia |
.334 |
.311 |
.324 |
wOBA |
Zach Davies |
.316 |
.295 |
.323 |
wOBA |
Aaron Nola |
.306 |
.286 |
.293 |
wxOBA |
**minimum 1,500 total pitches in both 2016 and 2017
Reviewing two full seasons of data tells a completely different story about the predictive power of wOBA…or lack thereof. Only three of the 10 pitchers in Table 4 came closer to their 2016 xwOBA in 2017 than to their actual 2016 wOBA.
Remember when I brought up FIP and BABIP at the beginning of this article? It’s frustrating to see people rushing headlong toward wxOBA as a Shiny New Toy because we have all been down this road before. In 2009, FIP was that shiny new toy. We were told, by many of the same people still plying their trade in this business, that FIP was all we needed to know about pitchers. FIP was – and still is – an interesting metric to look at when it comes to analyzing pitching, but it is merely a small piece of the puzzle, not the entire jigsaw.
This is likely the case for batted ball data, launch angles, wBA and xwOBA as well. These statistics are interesting to look at, and batted ball data can be fun and entertaining to hear about while watching a baseball game (some of my colleagues at Baseball Prospectus would vehemently disagree about the “fun and “entertaining” part). For now, it isn’t much more than that.
Beyond this, one challenge with using any metric for fantasy is that most fantasy games rely on traditional, old school stats that aren’t summarized tidily. Strikeouts are arguably the purest pitcher stat in traditional 5×5 Roto. Yes, catcher framing and umpire tendencies have some impact on strikeouts but over time, this tends to balance out. Every other statistic we use for pitchers in fantasy is flawed to one degree or another. ERA and WHIP rely on fielders and ballparks and don’t even get me started on wins and saves. Some of the challenge of playing fantasy baseball comes in recognizing that when you draft a pitcher, you’re not just drafting his skill set. You are also drafting his team’s defense, park effects and manager tendencies, among other things. Regardless of how useful a metric is, the great part about fantasy baseball is learning to incorporate all these factors into your analysis. It is messy, but as anyone who has played fantasy baseball for any appreciable amount of time knows, there isn’t a magic bullet that will solve this game any more than there is a one-size-fits-all statistic that will predict the future in the real-life version of it.
Thank you for reading
This is a free article. If you enjoyed it, consider subscribing to Baseball Prospectus. Subscriptions support ongoing public baseball research and analysis in an increasingly proprietary environment.
Subscribe now