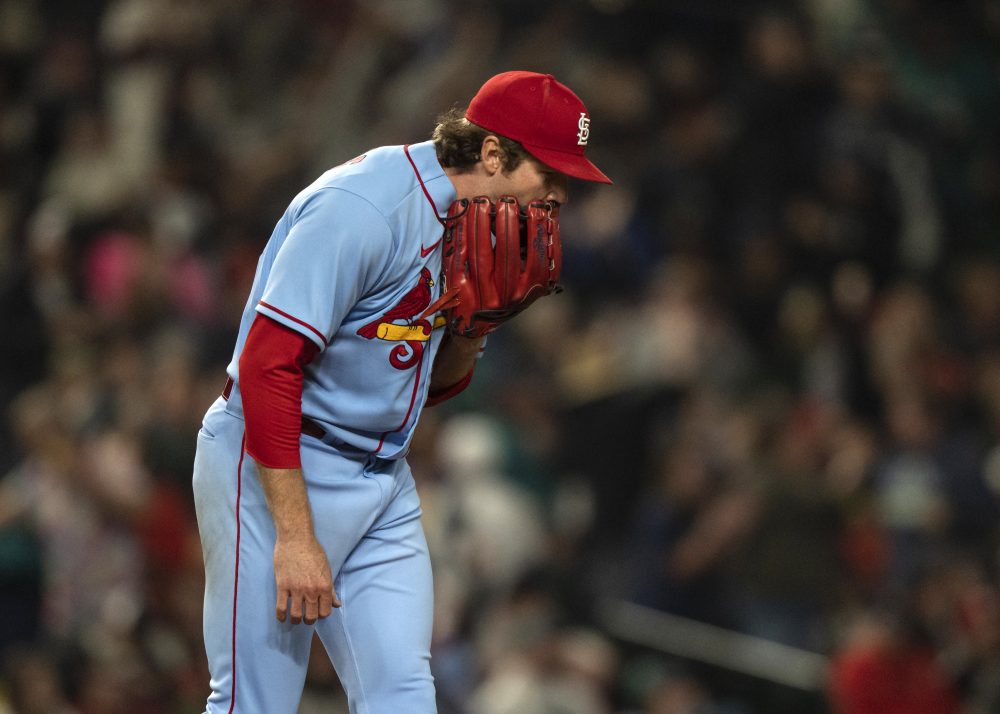
It’s been some time since we assessed the performance of popular pitching and hitting metrics. The continued integration of Statcast batted ball data has produced more competitive options for analysis. We are also seeing metrics focused on describing specific aspects of player performance, especially pitching, but which are also being promoted for prediction of player performance. As of this week, DRA and DRC, BP’s catch-all metrics to assess pitching and hitting, respectively, have been updated with Statcast inputs. In light of that update, we will evaluate their updated variants against other pitcher and hitter metrics, including certain “stuff” metrics.
We conclude that best-in-class, overall metrics are performing better than ever, but caution is necessary when using “stuff” metrics to predict future ERA. In particular, we find that while at least one “stuff” system’s ratings from 2021 correlated with pitcher 2022 ERA on the whole, this effect appears to be driven by pitchers who did not switch teams. This matters because a pitcher’s skill should be portable, and if a pitcher’s results depend on which team they are playing for, it is possible that it is team and park characteristics that are being measured, not the pitcher’s own skill. It is possible that connections between stuff metrics and pitcher ERA may be driven more by the way teams use the arsenals of pitchers than the arsenals of the pitchers themselves.
Background
Although the traditional measures by which baseball analysts measure player performance — the “slash line” for batters, and ERA for pitchers — are more useful than some are willing to admit, analysts have nonetheless spent decades trying to improve on their performance.
Publicly at least, these efforts for pitchers have included a fairly complex formula for better evaluating pitcher contributions, a much simpler one (Fielding Independent Pitching, or FIP), some attempts to improve on FIP (xFIP and SIERA), and more recent metrics like DRA.
Similar efforts for batters include On-Base-Plus-Slugging (OPS), which adds two out of the three triple slash values together, with surprising effectiveness; weighted on-base average (wOBA), which scales most of the available batting event outcomes onto one pseudo-binomial scale typically applied to hitters more than pitchers; and park-adjusted variants of those metrics like OPS+ and wRC+, respectively.
After the release of the Statcast system, Major League Baseball released “expected wOBA” (xwOBA), which divided wOBA into the two categories of what I distinguish as “not in play” events and “ball-in-play” events (“NIP” and “BIP,” respectively). For NIP events, xwOBA assumes that their raw values accurately measure the contributions of batters and pitchers, and accepts those values without adjustment. For BIP events, xwOBA assigns a wOBA value based on the average BIP outcome predicted by some combination of the ball’s launch angle and speed off the bat, with adjustment for the batter’s running speed for certain BIP types.
BP has developed its own metrics over multiple generations. In 2016, after introducing contextual FIP (cFIP), we then introduced Deserved Run Average (DRA or DRA-), which attempts to isolate the pitcher’s most likely contribution by controlling for park, quality of opponent, and by applying a general principle of skepticism to all outcomes, whether in play nor not in play. DRA has considered multiple additional factors over the years, but recently it has stuck to more basic inputs: play participants, park, platoon, starter vs. reliever, and home / away being the primary ones. DRA’s primary goal was to perform better than FIP (otherwise, why bother?), and by our measures, it has consistently done so.
After DRA began to mature, we moved into the hitting space with our analogous hitting metric, Deserved Runs Created (DRC+). DRC is the mirror image of DRA, using similar models, awarding hitters credit for outcomes only after considering the circumstances, and only then after seeing a consistent track record for each batting event type. When DRC was released, some commentators felt that the release of an updated hitting metric was unnecessary. As DRC’s performance numbers indicated, however, traditional metrics like OPS+ and wRC+ were giving batters too much credit for their box scores and not enough credit for their actual likely contributions. Bottom-line results do matter, but they are not the same thing as batter contributions to those results.
In reviewing this history, a few trends stick out.
Event Consolidation and Smoothing
First, especially with pitchers, there has been a trend toward ignoring or smoothing over events not believed to offer individual value. FIP does this most famously, counting only a pitcher’s NIP events (sometimes HBP is included, sometimes not) and one BIP event, home runs, in its calculation. Although FIP is often described as focusing on events “the pitcher can most control,” this is wrong. FIP’s focus is on the batting events that are “fielder independent,” and nothing more. By pleasant coincidence, those events also feature some of the largest run consequences, due to a combination of frequency and run value. Of those events, some are the events a pitcher most controls (strikeouts and walks). Pitchers have limited ability to control home runs, but if you take home runs out, you end up with kwERA, a nifty variant that describes pitcher skill but is less useful in explaining how runs get scored. From the other direction, xFIP leaves the NIP events alone but substitutes the league-average home run rate for a pitcher’s fly balls. This is an improvement in better describing a pitcher’s likely contribution, but also odd in its continued rejection of singles, a BIP category over which pitchers have substantial control, and which are both more valuable and more frequent than walks.
Rather than picking and choosing among events, other metrics ignore them entirely. SIERA is a linear regression to ERA, treating events as rate predictors of an overall ERA outcome, such that strikeout rate is directly considered along with ground ball rate. Although SIERA had a bit of a rough start (most new metrics do), it has continued to work well on the whole despite seemingly dated coefficients. xwOBA does this also. It is more straightforward to create a regression or machine learning model, toss a bunch of known-to-be-useful predictors into the soup, and find the seemingly-best combination of inputs to predict the expected output. DRA also did this at first, but the edges were quite sharp, as indicated by temporary DRA darling Jason Schmidt. We abandoned this “smooth over everything” approach soon afterward, in favor of a focus on predicting actual batting event outcomes, and aggregating the run value consequences of those outcomes.
DRA and DRC thus distinguish themselves in their (quaint?) insistence on modeling every individual batting event deemed to be interesting: strikeouts, walks, hit-batsmen, infield-reached-on-error, single, double, triple, and home runs. This approach is more difficult, in part because of the risk of overfitting those events. But this extra effort gives DRA and DRC an advantage: they can tell you why a pitcher or batter is being rated so poorly, whether it be because of their strikeout or double or home run rates. As a result, readers can go to our player cards and find out where a player is being dinged and why: separate deserved rates and/or run values for each batting event tell you what DRA / DRC see as each player’s strengths and weaknesses, relative to average, and helps you understand why these metrics reach the conclusions that they do.
For example, you can see that Christian Yelich has gone from an above-average singles and doubles hitter to a below-average singles and doubles hitter since he joined the Brewers in 2018, and that this trend has been constant from his transition to MVP and back again. During that MVP phase he went from a somewhat below-average home run hitter to a massively above-average one, and now back down to a below-average one. The runs contributed from walks, however, have trended more and more positive from the start, and this is one of the “old man skills” that still gives him value.
Statcast
The availability of directly-measured batted ball data during the 2015 MLB season was a revelation. In addition to allowing fans to better appreciate the inputs behind home runs, the measurements filled in a new part of the causal chain between play participants and play outcomes. With Statcast, analysts could now categorize hits into typical versus atypical outcomes, and puzzle out whose results were more deserving than others. Statcast does not solve all of our ball-in-play problems, because the batted-ball measurements are themselves outputs, not inputs, and treating batted-ball measurements as equivalent to skill is risky, especially for pitchers. To evaluate a player’s most likely contribution, we need to evaluate the player’s role in creating those batted ball measurements, not just assume players are completely responsible for what those measurements turned out to be.
The most prominent avatar of this new Statcast era is the aforementioned xwOBA. The metric’s early efforts were a bit rough, as again is often the case with these things. Shortly after its introduction, we advised that for pitchers, xwOBA was performing no better than FIP, and not as well as DRA. Likewise upon DRC’s introduction, we observed that while DRC and xwOBA were in a class by themselves for batters, more accurate than other metrics, DRC was still displaying better performance than xwOBA in the benchmarks we considered to be relevant. Up until now, DRA and DRC have not incorporated Statcast inputs, in part to avoid further complication, but also because it was not seen as necessary.
Of course, all things eventually change, and recently it became clear that xwOBA has made major strides since we last evaluated it. The improvement may be due to some combination of these changes discussed by Sam Sharpe along with the additional accuracy afforded by the Hawkeye system MLB began using in 2020. Whatever the cause, it is clear that xwOBA is notably more accurate (by our measurements) than it used to be, and the consequences of that improvement deserve both recognition and fresh review.
These improvements to xwOBA mean that DRC and DRA could no longer sit on the sidelines of Statcast, and as of today they no longer will. Both metrics now incorporate Statcast inputs on the batting events where we have concluded said influence is constructive, namely home runs and sometimes singles. With that addition, both metrics jump back to the level of performance we prefer to see relative to other metrics. The mechanics by which Statcast measurements were imported will be addressed by a separate article. For now, rest assured that both our composite and split (e.g. platoon) DRA/DRC values now benefit appropriately from Statcast in seasons and leagues for which it is publicly available.
Hitter Metrics, Generally
When we introduced DRC+ in 2018, we commented that from our standpoint, two metrics performed far better for hitters than the others: DRC+ and xwOBA. This has not changed.
Our view remains that the proper goal of sports player measurement is to determine each player’s most likely contribution, not just their results. We already have a source of information for a player’s results, and it is called a “box score.” Likewise, we already have an established means of assessing probable player skill, and it is called a “projection system.” Assessing players for their most likely contribution, typically over the course of a season, sits between these two extremes: we respect outcomes, because they are what actually happened. But we recognize that outcomes are complicated, and they don’t perfectly reflect player skill. We are not trying to predict the future, but we expect players of similar skill to make similar contributions, on average, so we expect metrics to rate the same players similarly over time (the concept of “reliability” or “stickiness”). We can also expect, in situations where a desired outcome is clearly quantifiable, to better predict future outcomes for these players (“predictiveness”), because similar ratings should coincide with similar results, on average. We have also discussed the accompanying concept of “descriptiveness,” accurately describing same-season outcomes, but have struggled to find a consistent use for it.
To business. The 2021 and 2022 seasons were the first full seasons to unleash Hawkeye, and they best illustrate the likely differences in performance between hitter and pitcher metrics for the 2023 season and beyond. As was the case in our recent defensive comparison, we rate the various metrics on their reliability to rate the same players, here from 2021 to 2022, and then in their predictiveness in anticipating the 2022 results (here, OPS) of those same players with their 2021 metric rating. No minimum PA was imposed (part-time players are people too), and correlations were weighted by averaging PA across both seasons for each player. Lastly, as a stress test, we limited the comparison to players who switched teams at some point between 2021 and 2022, to make it harder for metrics to profit from defense or ineffective park adjustments. (You will see why this matters in a bit). Metrics were acquired from Baseball Savant, FanGraphs, or BP as appropriate.
Using a weighted Spearman correlation (which allows us to compare metrics on different scales), the metrics rate as follows:
Table 1: Hitting Metric Comparison
(2021-2022, team-switchers, weighted Spearman by averaged PA)
Metric | Reliability | Predictiveness |
DRC+ (updated) | 0.67 | 0.55 |
xwOBA | 0.67 | 0.55 |
OPS | 0.49 | 0.49 |
wOBA | 0.48 | 0.48 |
wRC+ | 0.47 | 0.46 |
Batter evaluation was a two-metric race a few years back and that has not changed; if anything, the gap has widened. Thus, when DRC+ or xwOBA are available, OPS, wOBA, or wRC+ (or OPS+, I suppose) should be used to summarize a hitter’s results, not their likely offensive contributions.
Despite their performance advantage, DRC+ and xwOBA can still display rough edges. xwOBA tends to favor players who hit the ball hard and in the air; your Jeff McNeil and Luis Arraez types of players are likely to be undervalued. The flip side is that, to the extent a player’s contributions are unusually driven by those two qualities, DRC+ may be less impressed than it should be, and not as quickly as it might be, even though it now considers many of the same inputs. As noted above, xwOBA also does not distinguish between individual batting event outcomes, so it is not able to report on which events (e.g., singles versus doubles) are happening more or less than expected. However, other metrics uniquely offered by MLB do call out factors like quality of contact that get at some of these issues, and offer more insight on others.
Pitching Metrics, Generally
We begin by comparing various public metrics on the twin measures of reliability and predictiveness, as we did with hitter metrics. Here, we judge predictiveness by RA9, rather than OPS, and weight the correlations by average IP across both seasons:
Table 2: Pitcher Metric Comparison
(2021-2022, team-switchers, weighted Spearman by averaged IP)
Metric | Reliability | Predictiveness |
DRA (updated) | .53 | .26 |
cFIP (updated) | .51 | .25 |
xwOBA / xERA | .39 | .23 |
SIERA | .46 | .18 |
kwERA | .48 | .19 |
xFIP | .44 | .19 |
FIP | .34 | .19 |
ERA | .13 | .10 |
DRA and cFIP, particularly with their Statcast updates, stand out to some extent. xwOBA has curiously meh reliability but manages to recover in predictiveness. xwOBA’s challenge is that it effectively assumes that pitchers and hitters are equally responsible for BIP launch angles and exit velocities, and this is not true. However, in its own way it holds its own, and it is certainly now a clear improvement over FIP. (MLB’s xERA is a scaled xwOBA that should produce the same result, so we do not consider it separately).
SIERA, kwERA, and xFIP do a nice job anticipating themselves, but the results are not there on the predictiveness side. This is curious as these metrics have done a nice job in the past; it’s possible that batter/pitcher approaches have changed in a way these metrics are not able to detect. As for traditional FIP, it comes in last in both categories. FIP’s low reliability as compared to other metrics belies the notion that FIP is describing those events “a pitcher can control.” FIP certainly does not claim to be predictive, but our view is that for a metric to accurately measure player contributions, as opposed to results, it must demonstrate this predictive power—and FIP is widely used to measure pitcher contributions.
Anticipating pitching results is certainly difficult, as this chart demonstrates. But by both reliability and predictiveness, DRA and cFIP remain strong choices by which to evaluate pitcher contributions.
Pitcher Stuff Metrics
Pitcher “stuff” metrics have become popular recently, trying to go beyond a pitcher’s results to better understand the driving forces of those results. In general, the breakdown tends to be a pitcher’s raw pitch characteristics (aka “stuff”) as separated from strike zone location, and some effort to combine the contributions of those two. The overall approach makes sense, at least at a high level.
Pitchers of course tend to have multiple pitches, and each of their pitches have various distinguishing aspects—multiple directions of movement, velocity, release points, and approach angles, among others—that combine in varying ways to provide an effective pitching arsenal. Summarizing these multiple characteristics with one or at least only a few numbers is desirable, but collapsing multiple inputs into few or one is hard to do, because these inputs derive their value in combination with other inputs. Velocity might be the closest thing to an input that stands on its own, but fastballs down the middle of the plate are often dangerous regardless of speed, and pitcher who can do other things are generally going to be more successful.
Typically employing some type of boosted or bagged-tree approach, “stuff” modelers seem to hone in on combinations of inputs that their models see as most consistently effective. They pair those inputs with some combination of desired outcomes (whiffs, exit velocity, launch angle, etc.), as well as the run values of those outcomes, to produce a composite score that rates these different aspects of pitching: the “stuff,” the “location,” and then sometimes a composite of these composites to give a final overall grade.
The most prominent variant of these “stuff” metrics is called, appropriately, “Stuff+” and is available from our friends at FanGraphs. Technically, the Stuff+ system seems to have three parts: Stuff+ (pitch characteristics), Location+ (self-explanatory), and a third measurement of Pitching+ that combines the two into an overall output. FanGraphs also publishes a competing system by PitchingBot.
Fans of Stuff+ stress two claimed advantages. First, they assert that it “stabilizes” quickly. Second, Stuff+ is claimed to be more predictive of ERA than traditional ERA/RA9 estimators, despite working with only a subset of the same information. We will address both claims in turn.
Stuff + Reliability
I don’t like “stabilization” analysis or anything to do with Cronbach’s Alpha, but the underlying point is the same as Reliability: the metric effectively predicts itself, meaning that it rates similar performances similarly over time. This is an important component of a useful metric, and I agree that Stuff+ is quite reliable. Using the same dataset we used above:
Table 3: Stuff+ Metric Reliability
(2021-2022, team-switchers, weighted Spearman by averaged IP)
Metric | Reliability |
Stuff+ | .74 |
Location+ | .62 |
Pitching+ | .59 |
These reliability numbers are higher than those for the ERA / RA9 estimators in Table 2, and it should be clear why that is: Stuff+ and Location+ are isolating specific sub-components of a pitcher’s results. Those sub-components of course tend to be consistent for individual pitchers: pitchers tend to throw with similar velocity and to throw similar pitches from year to year. Additionally, pitchers with good control tend to continue to have good control, although somewhat less consistently than pitchers who tend to throw the same underlying pitches, whether they locate them or not. So while high reliability is a good thing to see, it is also something we expect to see.
Stuff+ Predictiveness
Stuff+ ERA prediction assessments typically rely on attempts to craft a projection system based on multiple seasons of Stuff+ ratings. I have concerns about this approach, but the bottom line isn’t that different from what we have been doing in this article: Either you successfully anticipate the success of pitchers from year to year or you do not. So we will proceed to evaluate Stuff+ for predictiveness, as we have the other pitcher run estimators above. Because we only have two full seasons of data, we will test the ability of 2021 Stuff+ measurements to predict 2022 pitcher ERA.
And when we do this, we notice something that is both curious and concerning. Stuff+ enthusiasts are correct that Stuff+ metrics predict ERA, in a manner of speaking. But, the ERA being predicted appears to only be partially attributable to the pitcher and his arsenal.
Consider this next table, in which we again compare ERA prediction accuracy, but do so over three cohorts: (1) pitchers who stayed with the same team between 2021 and 2022; (2) all pitchers who pitched in 2021 and 2022; (3) pitchers who switched teams at some point in 2021 or 2022.
The reported correlation values to next year’s ERA are nearly identical to those for predicting RA9, which is what we used above, but we will use ERA here because it has been the object of comparison for public discussions of stuff metrics:
Table 4: ERA Prediction, DRA vs. Stuff Metrics, by Pitcher Status
(2021-2022, weighted Spearman by averaged IP)
Metric | Same Team | All Pitchers | Switched Teams |
Stuff+ | .41 | .33 | .14 |
Location+ | 0 | .09 | .24 |
Pitching+ | .35 | .31 | .23 |
DRA (updated) | .32 | .30 | .27 |
(Note that in making these calculations we just used the absolute distance away from zero because “plus” metrics rate good performance in the opposite direction of ERA. This doesn’t affect the validity of values). We also include DRA because it was the best-performing pitcher run estimator above.
In our write-up introducing Range Defense Added (RDA) a few months ago, we stressed the importance of subjecting metrics to a “severe test,” to quote Deborah Mayo. A severe test is one that makes it difficult to succeed for any reason other than a valid one: truly good performance in the measurement of choice. For us, that meant we graded defensive metrics exclusively on players who had switched teams, rather than all players. As we explained:
Fielder ratings should not be getting polluted, even inadvertently, from the quality of their team’s positioning decisions or neighboring fielders. The cleanest way to take these confounders out of the system is to rip the band-aid off, and evaluate your metric on its ability to correctly rank, year after year, the best and worst fielders who have been shipped off to other teams.
We have applied that same principle throughout this article. Thus, in our master pitching table above, Table 2, we exclusively used team-switchers to compare metric performance. DRA’s progression in Table 4 reflects what you also see from the other established metrics in Table 2: it performs best with pitchers who stay with their existing teams. You see a slight decline when going from pitchers who stayed with their teams to all pitchers, and then another slight decline when you move exclusively to pitchers who switched teams.
The changes we see in Stuff+ and its sister metrics are more concerning. Stuff+ has a strong relationship with ERA…as long as we look solely at pitchers who stayed with the same teams both seasons; otherwise, its predictive power vanishes. If the ERA being predicted was primarily driven by the inherent “stuff” of the pitcher, this should not be happening. When we switch to the severe test of team-switchers, Stuff+ becomes a poor predictor of ERA, worse than FIP.
Location+ has its own concerning change in predictive power. Location+ is quite predictive with team switchers but rates as utterly useless to pitchers who remain with their teams. Obviously, this cannot be right: location is important to all pitchers, whether it is with their current team or some other team they join. A pitcher who cannot find the strike zone is a pitcher out of a job, with his new team or his old team.
The strong reliability ratings for these metrics in Table 3 are also relevant here. From those ratings, we know that Stuff+ and its companion metrics are consistently giving the same guys similar ratings, and doing so to a higher degree than best-in-class ERA estimators. (Again, this is expected given their focus on pitch characteristics rather than pitch results). So the issue isn’t that Stuff+ sees these guys as having changed; rather they are being rated as the same guys, except that their ERA can no longer be predicted the moment they go to another team.
Of the various cohorts in Table 4, the ratings for team-switchers seem like the “correct” ones. Those scores suggest that locating pitches is more relevant to future ERA than the inherent quality of those pitches (which makes sense), and, conversely, that the quality of one’s pitches doesn’t matter much for run prevention if you can’t locate them. This also makes sense. There is a reason why Wade Miley has been around for a decade-plus while flamethrowers with poor control come and go. Finally, to the extent Pitching+ is designed to be a combo of Stuff+ and Location+, its score in between Stuff+ and Location+ makes sense as well.
But why is this disparity happening at all? One possibility is that the sample sizes are small and this is just noise. But the magnitude of these differences is large, and no other reputable pitching metric displays similar behavior. The sample is also not that small: at least in our groups, we have 231 pitchers who pitched for more than one team and 342 pitchers who stayed put for 2021 and 2022. It’s not thousands of pitchers, but it’s not 30 pitchers either. When we took 5,000 bootstrap samples of these hundreds of rank correlations, the standard deviation of the overall average correlations was .05–.06 for players who stayed with their teams and .06–.07 for players who left. That puts the most extreme correlation changes in Table 4 at or outside a 95th percentile confidence interval. Could it be a coincidence? I suppose. Is it likely? Not really.
The most straightforward reason a metric would strongly predict ERA for pitchers who stayed with their team and poorly with pitchers who left would be that the metric is tracking the pitcher’s team and that team’s run environment, not (just) the pitcher himself. In fact, this is one reason ERA performs well predicting the second half of a pitcher’s current season but poorly predicts a pitcher’s next season: ERA is strongly influenced by the parks that are pitched in and the quality of fielders behind each pitcher. A lot (more) can change over one offseason.
But why would that be happening? My understanding is that Stuff+ and metrics like it focus on abstract skills like whiffs and Statcast batted ball measurements, which in theory should resist these problems, and in fact are chosen under the belief that they should resist these problems. But the numbers speak for themselves, and they are compelling. Furthermore, if Stuff+ displays this type of relationship with ERA, it’s likely that other stuff metrics could display similar infirmities.
We don’t purport to know the entire answer, but we have some hypotheses about what might be going on.
First, it is likely that existing pitcher run estimators capture much of the information available to be had from NIP events, because, unlike other events in baseball, strikeouts and walks require multiple pitch outcomes to be realized: three strikes for strikeouts, four balls for walks. I call these composite batting events for this reason. Thus, it is difficult to consistently strike out or walk batters by accident, and these statistics largely speak for themselves in fairly crediting a pitcher for their results. xwOBA, as we noted above, makes this very assumption; while DRx and cFIP model those events further, the additional gains are somewhat modest. As a result, stuff metrics may have little additional to offer with NIP events and may present a substantial risk of offering less.
That leaves BIP metrics as an area for performance differentiation, and BIP events are far more difficult to separate from the strategy decisions made by teams and the quality of fielders a team provides. Teams with great defenses might be less worried about the nature of balls a pitcher allows to be put into play. Different teams will favor different pitch combinations, particularly in certain situations, and stuff metrics may find some pitch types easier to grade consistently.
Second, somewhat related to the first point, teams often adjust the relative usage of pitches in a pitcher’s arsenal, or at least ways in which those pitches are used. Perhaps the new team has a “philosophy” about which pitches they like and when they like them. Because pitches of course play off one another, this could have cascading effects, particularly if the new philosophy is a tough match to the pitcher—at least at first.
Finally, using launch angle and exit velocity as proxies for batted ball quality risks making the same assumption we warned about earlier: that pitchers are fully responsible for the launch angles and launch speeds they permit. At a minimum, for launch speed, we know that is just not true. If one looks at launch speed in isolation, batter identity arguably explains four to five times the variance in exit velocity than pitcher identity does. Of course, here we are speaking of opponents, not teammates, and opponents to some extent wash out. But pitchers on the same teams tend to face a similar mix of batters, particularly within the division, and pitchers who move to other teams or leagues may face a somewhat different mix.
None of these explanations is fully satisfying, individually or taken together. One conclusion, of course, would be that Stuff+ and other metrics of its sort should not be getting used to predict ERA at all; instead, they should be satisfied to function as sufficient statistics for describing a pitcher’s overall pitch quality, which is a useful thing all by itself. But better understanding the connection between arsenal metrics and pitcher results also seems like an incredibly useful thing, and this review suggests the community still has a ways to go to get there.
Thank you for reading
This is a free article. If you enjoyed it, consider subscribing to Baseball Prospectus. Subscriptions support ongoing public baseball research and analysis in an increasingly proprietary environment.
Subscribe now
I'm trying to assess DRA- for various pitchers using inning limits in the denominator eg best 2000, best 3000, best 10 yr best 5 yr, so weighting the annual data based on innings matters. Is this a mistake -using innings rather than PA or BF? Are the annual DRA- as noted on the site [searchable by year] accurate?
My one question: In reviewing DRA-, I get some surprises.
Example 1: Jim Bunning's DRA when calculated by summing annual numbers eg [ innings in given year / total career innings ] x DRA- for that year, I get a far different number than career DRA- as published also on BP. Career DRA- =87 based on Beyond the box score Romano 2/29/2016 but 78 by summing years based on IP with BP data.
Anyway, fascinating stuff