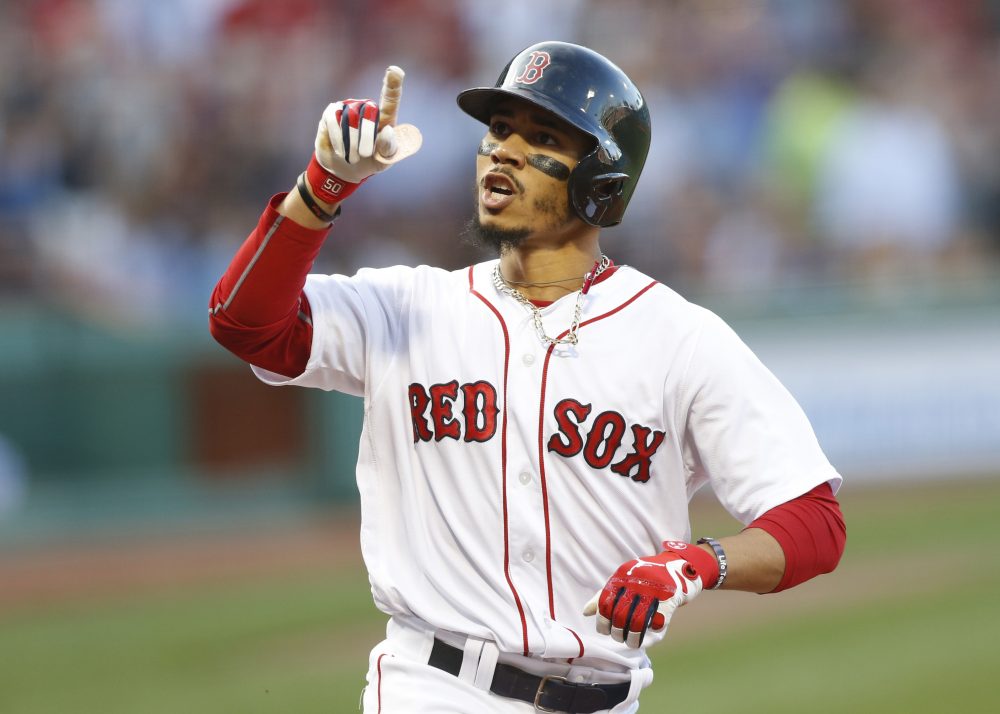
This article is part of the launch for Baseball Prospectus’ new hitting statistic, Deserved Runs Created, which you can learn much more about here.
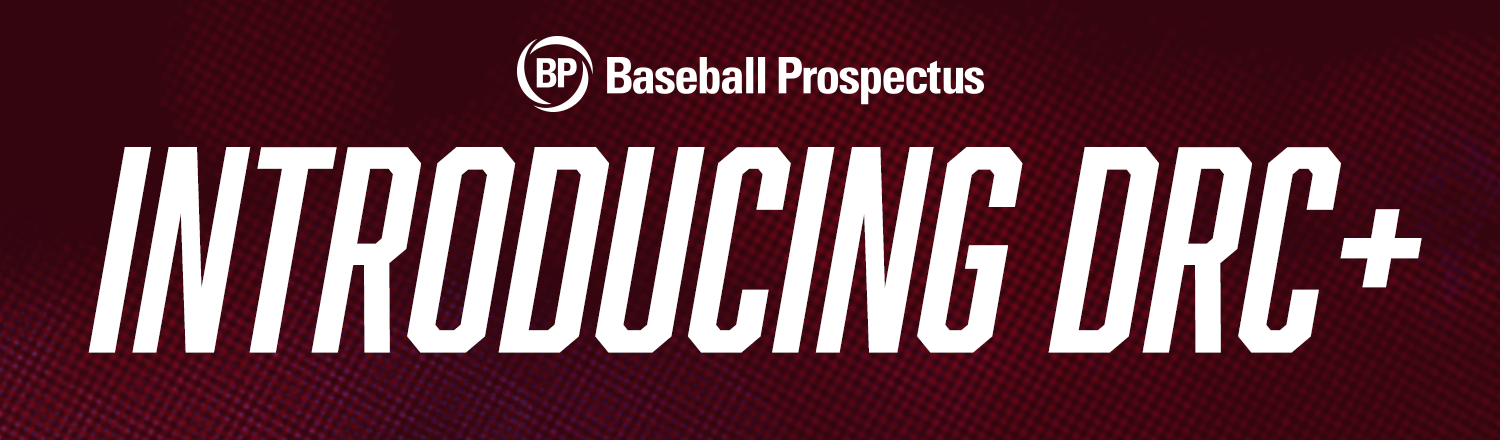
One of our statistical themes this year has been batting offense. We started by explaining the need for proper benchmarks and evaluated various Statcast metrics on their performance. We updated and revisited a debate between two offensive metrics, wOBA and OPS. We then discussed at length the concept of a player’s “expected contribution”[1] and how it underlays our concept of “deserved runs.”
Today, that progression reaches its peak with the introduction of Deserved Runs Created, which we will publish under the moniker “Deserved Runs Created Plus,” or DRC+. DRC+ is essentially the flipside of Deserved Run Average (DRA): it involves similar models and extractions but is designed and grouped to track batter performance rather than pitcher performance.
Park-adjusted offensive baseball statistics are often presented on a “100” scale using a “plus” moniker that indicates higher values are better. Thus, just as we have DRA- as our scaled-to-100 statistic for pitchers (lower is better), we now have DRC+ as our scaled-to-100 statistic for hitters (higher is better). DRC+ exists for any season with reliable play-by-play data, from 1921 to the present.
Why use DRC+? The simple answer is because it’s more accurate than wRC+, OPS+, and True Average in every relevant way. The more urgent answer is that wRC+ and OPS+ appear to be compromised by the changing baseball, whereas DRC+ is much less affected. In sum, if park- and opponent-adjusted accuracy is important to you, then DRC+ should be your offensive metric of choice.
The Superior Accuracy of DRC+
In August, we laid out the underlying principles of our search for the “expected contribution” for players, and why virtually all traditional leaderboards fail to accurately report these contributions. Those principles included (1) that the fundamental unit of player measurement was seasonal, (2) that a player’s expected contribution cannot be directly observed, and (3) that a review of three so-called Contribution Measures—reliability, predictiveness, and descriptiveness, in that preferred order—seemed to distinguish the best estimates of past player contributions.
In an otherwise complicated analysis, we derived one easy rule, which we labeled Principle 7: If one metric outperforms another in all three Contribution Measures, it is almost certainly superior. This finding was justified both (a) by inference (only greater signal seemingly explains across-the-board superiority in inconsistent tests) and (b) by experience, given that generally-accepted superior metrics like wOBA and OPS exhibited this same quality relative to OBP and especially batting average.
Principle 7, then, could make this a very short article. The simple reason to prefer DRC+ is because, over the past several decades, it easily outperforms all competing park-adjusted hitting metrics in all three measures:
Table 1: Batting Metric Performance by Contribution Measures (teams, 1980–2018); Robust Pearson Correlation to Team Runs/PA
Metric | Rel | Rel_Err | Pred | Pred_Err | Desc | Desc_Err |
DRC+ | 0.63 | 0.02 | 0.42 | 0.03 | 0.72 | 0.02 |
True Average | 0.54 | 0.02 | 0.34 | 0.03 | 0.67 | 0.02 |
wRC+ | 0.54 | 0.02 | 0.35 | 0.03 | 0.69 | 0.02 |
OPS+ | 0.50 | 0.02 | 0.35 | 0.03 | 0.68 | 0.02 |
This table is similar to the table for raw offensive metrics we published back in August, except this time we (1) are showing park-adjusted metrics, which most analysts prefer because they attempt to neutralize stadium effects, and (2) have included the most recent season, which has since completed.
Like last time, the comparisons are robust Pearson correlations, compiled at the team level, between team averages for these metrics and team runs scored, and higher is better. The performance of park-adjusted metrics is not as good as the raw metrics we saw in August, but this makes sense, given that park-adjusted metrics assume many actual runs scored were undeserved, placing a ceiling on the extent to which such metrics will ever correlate with raw run rates. Nonetheless, testing to raw runs is still useful, as we still want to see any metric, even a park-adjusted one, adhere as much to reality as possible after its adjustments.
On average, over the past several decades, DRC+ is clearly the most accurate park-adjusted metric. It is much more reliable than wRC+ and OPS+, scoring well beyond the margin of error[2] for both. This is because DRC+ is doing a better job of deriving actual player contributions than wRC+ or OPS+. DRC+ also has notably better predictive performance than the other metrics, with its superiority in that respect also outside the margin of error. The lead for DRC+ continues in descriptiveness, which we consider to be the least important of the three measures but here is consistent with the other findings.
We suspect that the gulf in quality between DRC+ and these other metrics is somewhat masked by the comparison to raw team runs, because again there is only so much unadjusted run-scoring for which an adjusted metric can account. Let’s look at some comparisons that specifically test the power of a metric to adjust for different environments, which is where a park-adjusted metric should thrive. This also allows us to focus on individual variation rather than team averages. We’ll put the raw, unadjusted metrics back in for comparison also.
Specifically, let’s look at individual players who switched teams in consecutive years, thereby moving themselves into another run-scoring environment for half of their games. For reasons that will become clear shortly, we’ll also limit ourselves to a collection of more recent seasons:
Table 2: Reliability of Team-Switchers, Year 1 to Year 2 (2010-2018); Normal Pearson Correlations[3]
Metric | Reliability | Error | Variance Accounted For |
DRC+ | 0.73 | 0.001 | 53% |
wOBA | 0.35 | 0.001 | 12% |
wRC+ | 0.35 | 0.001 | 12% |
OPS+ | 0.34 | 0.001 | 12% |
OPS | 0.33 | 0.002 | 11% |
True Average | 0.30 | 0.002 | 9% |
AVG | 0.30 | 0.002 | 9% |
OBP | 0.30 | 0.002 | 9% |
With this comparison, DRC+ pulls far ahead of all other batting metrics, park-adjusted and unadjusted. There are essentially three tiers of performance: (1) the group at the bottom, ranging from correlations of .3 to .33; (2) the middle group of wOBA and wRC+, which are a clear level up from the other metrics; and finally (3) DRC+, which has almost double the reliability of the other metrics.
You should pay attention to the “Variance Accounted For” column, more commonly known as r-squared. DRC+ accounts for over three times as much variance between batters than the next-best batting metric. In fact, one season of DRC+ explains over half of the expected differences in plate appearance quality between hitters who have switched teams; wRC+ checks in at a mere 16 percent. The difference is not only clear: it is not even close.
Let’s look at Predictiveness. It’s a very good sign that DRC+ correlates well with itself, but games are won by actual runs, not deserved runs. Using wOBA as a surrogate for run-scoring, how predictive is DRC+ for a hitter’s performance in the following season?
Table 3: Reliability of Team-Switchers, Year 1 to Year 2 wOBA (2010-2018); Normal Pearson Correlations
Metric | Predictiveness | Error |
DRC+ | 0.50 | 0.001 |
wOBA | 0.37 | 0.001 |
wRC+ | 0.37 | 0.002 |
OPS+ | 0.37 | 0.001 |
OPS | 0.35 | 0.002 |
True Average | 0.34 | 0.002 |
OBP | 0.30 | 0.002 |
AVG | 0.25 | 0.002 |
If we may, let’s take a moment to reflect on the differences in performance we see in Table 3. It took baseball decades to reach consensus on the importance of OBP over AVG (worth five points of predictiveness), not to mention OPS (another five points), and finally to reach the existing standard metric, wOBA, in 2006. Over slightly more than a century, that represents an improvement of 12 points of predictiveness. Just over 10 years later, DRC+ now offers 13 points of improvement over wOBA alone.
Our existing offensive baseline at BP, True Average, while competitive, fails to stand out. Thus, while True Average will still be selectable in our Sortable Stats tables, it will be retired as the default batting metric of Baseball Prospectus. Batting Wins Above Replacement Player (BWARP) will instead be calculated from Deserved Runs Above Average (DRAA), which in turn are derived from same process culminating in DRC+. True Average is, for all practical purposes, no more.
DRC+ and the Altered Baseball
Ordinarily, the introduction of a new metric merely gives analysts another option to consider. Here, however, we think the situation is a bit more urgent. Put simply, the changing baseball has wreaked havoc on traditional park-adjusted metrics. Thus, if you wish to use wRC+ or OPS+, particularly to study the last several baseball seasons, we recommend that you do so only with extreme caution.
In Table 1 above, we showed you correlations for park-adjusted metrics for all years from 1980 through 2017. Let’s return to team-level numbers but focus now on the 2010-2018 seasons.[4] This is a brutal sequence of years for park-adjusted metrics: baseball’s run environment plunges to just over 4.0 runs a game in 2014, and then rebounds a few years later to 4.5 runs per game. Furthermore, between 2015 through 2017, it is now admitted that the baseball physically changed, perhaps multiple times, causing run-scoring to rebound even though many of the structural factors that coincided with the earlier decline in run-scoring—more strikeouts, higher velocity, and increased pitcher substitution—remained present.
It is bad enough to have run-scoring going back and forth from season to season; it’s even worse to have, as a backdrop, the baseball itself changing, at varying rates, which could affect different players and ballparks differently. Let’s see how our park-adjusted metrics do from 2010 onward:
Table 4: Batting Metric Performance by Contribution Measures (teams, 2010–2018); Robust Pearson Correlation to Team Runs/PA
Metric | Rel | Rel_Err | Pred | Pred_Err | Desc | Desc_Err |
DRC+ | 0.63 | 0.02 | 0.42 | 0.03 | 0.72 | 0.02 |
wRC+ | 0.43 | 0.06 | 0.28 | 0.07 | 0.66 | 0.04 |
OPS+ | 0.37 | 0.07 | 0.27 | 0.07 | 0.66 | 0.05 |
The error rates are higher because the number of seasons is smaller. Nonetheless, the results are clear, even in this “stress test” situation. Once again, DRC+ is superior to the other park-adjusted metrics in every way, and well outside the margin of error on the two metrics that matter the most: Reliability and Predictiveness. More importantly, compared to Table 1, which showed all seasons from 1980-2018, DRC+ experiences no dropoff whatsoever in performance, despite the changing ball and volatile run environment. wRC+ and OPS+, on the other hand, experience notable declines in their reliability and predictive power, with descriptive power somewhat suffering also.
Why does DRC+ perform so much better, both on average and during times of statistical stress? We can think of (at least) three reasons.
The first is that DRC+, unlike other park-adjusted batting metrics, is designed to look behind the numbers for the player’s expected contribution, rather than take play outcomes otherwise at face value like wRC+ and OPS+ do. Virtually all outcomes, good or bad, get shrunk to incorporate skepticism and award only partial credit for each play. The effect of this is particularly notable on home runs, which can unreasonably dominate the values of other metrics. Batters without question deserve much of the credit for home runs they hit, and DRC+ certainly gives them plenty. But by recognizing that other factors are often at play, DRC+ avoids letting home runs essentially rule the roost, allowing other (and more stable) accomplishments like walk and strikeout rate to play a more prominent role.
The second is that DRC+ is adjusted to account for more factors, most notably quality of opponent. I generally would classify these adjustments more as “nice to have” than critically important, but in the face of such drastically different performance it is reasonable to consider the possible impact of these additional adjustments also.
But the third—and possibly most important—reason DRC+ is better is that it uses single-year park factors rather than the multi-year park factors favored by the other metrics in widespread use. Traditionally, multi-year park factors have been viewed as more stable and less influenced by one-year blips; the flipside is that when the one-year changes are meaningful, the multi-year park factor can miss the change and possibly credit/penalize players in the wrong direction over multiple years. Sabermetric writer Patriot has a wonderful discussion of the tradeoffs here.
In that respect, the altered ball situation may have created a bit of a perfect storm. The extent to which the altered ball has affected parks differently has not been publicly explored in detail, but suffice it to say that the yo-yo run environment has put multi-year park factors in a difficult position. An approach that made sense for years now may be in crisis.
A compromise would be to use single-season park ratings, but to incorporate some skepticism (there’s that word again) into the responsibility assigned to each stadium. That is the approach taken by the models underlying DRA and DRC+, although they don’t use park “factors” per se. Park factors are previously-calculated inputs to metrics like wRC+ and OPS+; for the deserved-run models, parks (controlled for platoon) are just additional variables in the various regressions for each component.
However, we can extract the overall effects of those variables to create a “park rating” of sorts by DRC+ for the various stadiums. On a 100-scale, with 100 being average, and combining the platoon splits, the park ratings for 2018 extract as follows:
Table 5: Net Park Ratings, 2018
Stadium | Park Rating[5] |
COL | 104.0 |
CIN | 103.0 |
PHI | 102.0 |
BOS | 101.8 |
TOR | 101.7 |
MIL | 101.6 |
TEX | 101.5 |
NYA | 101.4 |
CLE | 101.1 |
WAS | 101.0 |
SDN | 100.7 |
CHA | 100.6 |
CHN | 100.5 |
LAN | 100.5 |
MIN | 100.5 |
ARI | 100.4 |
PIT | 100.1 |
SEA | 100.0 |
ANA | 99.8 |
SFN | 99.7 |
BAL | 99.5 |
HOU | 99.4 |
TBA | 99.4 |
DET | 99.3 |
ATL | 99.2 |
KCA | 99.2 |
NYN | 98.8 |
OAK | 98.6 |
MIA | 98.2 |
SLN | 98.1 |
You’ll note that the hierarchy of parks is roughly what you might expect, with perhaps some surprises; what may surprise you more is how limited the probable effect of the parks is found to be, even over only one year. By tracking the run-scoring effect of a park on a per-season basis, but keeping those values from getting too extreme, the “deserved” family of metrics can react each season to changes in a park or the baseball, without overreacting to any such changes. This is not the only way to do it, but it is an approach that seems to work, as verified by the correlation performances reported above.
Lastly, we will compare the performance of DRC+ to Statcast. Statcast offensive performance is typically reported by what MLBAM calls xwOBA, and somewhat less commonly by what it calls xBA. Neither statistic appears to be easily available grouped by player and team, but it is available grouped by individual and offers the ability to compare either statistic to a player’s current or next year wOBA, as well as the next year of the same statistic. So, comparing reliability (correlation to next year’s measurement for same individual), predictiveness (correlation to next season’s wOBA) and descriptiveness (correlation to same year’s wOBA), here are the comparisons between DRC+ and Statcast metrics, over the four years the latter has been in existence:
Table 6: DRC+ vs. Statcast by Contribution Measures (individuals by total season, Gaussian correlations, 2015–2018)
Metric | Rel | Rel_Err | Pred | Pred_Err | Desc | Desc_Err |
DRC+ | 0.77 | .001 | 0.59 | 0.001 | 0.88 | ~0 |
Statcast xwOBA | 0.63 | .001 | 0.52 | 0.001 | 0.87 | ~0 |
Statcast xBA | 0.56 | .001 | 0.42 | 0.001 | 0.75 | 0.001 |
Statcast metrics have the advantage of superior inputs, but those inputs appear to have limitations also. Both xwOBA and xBA are trounced by DRC+, largely across the board. And unlike Statcast, DRC+ offers superior accuracy not just from 2015-2018, but all the way back to 1921.
Conclusion
We will have much more to tell you in the coming weeks about DRC+, along with a discussion of how the models work and why they work so well.
In the meantime, we are delighted to unveil the culmination of several months of work, and hope you find it useful as you seek to better understand the probable contributions of baseball hitters at the plate.
Many thanks to the BP Stats team for extensive peer review and discussion.
[1] As originally posted, the paper spoke of the “most likely contribution.” After a late-night talk with Stan developer Jonah Gabry, I agree that the better term is “expected contribution,” in the sense that we are looking for an overall “expectation” of past performance, not the most likely such performance. The “expected” contribution should not be confused with the “x” or “expected” concept in terms of predicting future performance, which is unfortunately well-established in the fantasy world, but also potentially confusing in this context.
[2] As in our article comparing wOBA and OPS, we are using brms and having it report the probable correlation between each metric and team runs/PA as multivariate outputs using Markov Chain Monte Carlo sampling. Error rates are calculated by brms / Stan along with the reported correlation coefficients.
[3] We have switched to normal Pearson correlations to accommodate outliers; if you use robust (student’s t) correlations, as in Table 1, the order remains the same although the values compress somewhat. We are otherwise using the same brms / Stan procedure referenced above.
[4] 2010-2017 for descriptiveness, with 2018 added to provide the necessary second year for reliability and predictiveness.
[5] Park ratings are based on the raw regression coefficients for each event, as separated by handedness, in their raw form. These composite “park ratings” combine the net run effect for each park of each modeled event type, by handedness, into one overall rating per park.
Thank you for reading
This is a free article. If you enjoyed it, consider subscribing to Baseball Prospectus. Subscriptions support ongoing public baseball research and analysis in an increasingly proprietary environment.
Subscribe now
I would imagine that xwOBA would benefit greatly from quality of opponent and park factor adjustments. As you mention, the superior inputs give it a huge advantage.