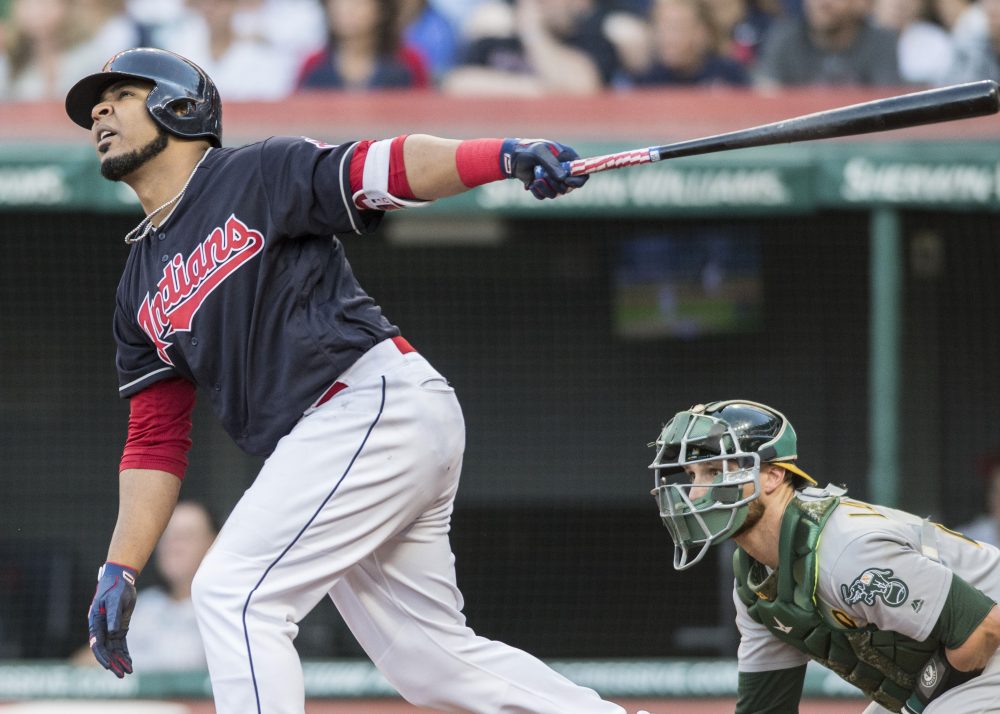
We continue to scrutinize and update our metrics, and as part of that process we’ve been comparing various offensive metrics to one another.
Two of the metrics we’ve checked in on are weighted On Base Average (wOBA), popularized by Tango et al in The Book in 2007, and On-Base-Plus-Slugging (OPS), a statistic popularized by The Hidden Game of Baseball, published by Pete Palmer and John Thorn in 1984. Because comparisons between these two have a bit of a history, I thought we would start this series by updating those comparisons.
Some Brief Background
OPS is straightforward, at least in concept. You take a batter’s on-base percentage (OBP)—which admittedly is not much of a percentage—add it to their slugging percentage (SLG)—definitely not a real percentage (baseball stats can be very strange)—and the sum of those two numbers gives you the “OPS.”
wOBA is more complicated. wOBA assigns “linear weights” to various baseball batting events; linear weights are the average number of runs scored in a half-inning after such an event occurs. For wOBA, those run values are then re-scaled to put them on the same general scale as OBP, which means ensuring that all outs equal 0. This additional scaling is not necessary, but the authors of The Book thought it would be useful (or at least more persuasive) to have OBP and wOBA on the same scale.
Those who have read The Book know that the authors are not impressed by OPS: they complain that OBP and SLG have overlapping components, different denominators, and that OPS substantially under-credits the importance of OBP. In other words, the authors of The Book view OPS as an approximation at best, useful only as a “gateway” statistic, if that. In their view, analysts focused on accuracy ought not to be using OPS.
Which Metric is “Better”?
With that introduction, let us go back five years to a post that started an interesting discussion.
In July 2013, Cyril Morong, an economics professor at San Antonio College, wanted to compare the performance of OPS and wOBA in predicting run scoring. This is a tricky thing to do for individual batters, since unlike pitchers, there is no “run-generated” analogue to RA9. To get a defined pool of runs to work with, Morong went one level “up” to team run rates. Because all individuals are associated with a team when they bat, and the weighted average production of all team batters gives an overall OPS or wOBA for the team, we can instead look at the average team OPS or team wOBA and compare that to the average team runs scored per plate appearance.
When he did this, Morong found something interesting. Looking at all teams from the 2010-2012 seasons, he found that team OPS correlated slightly better to team run production rates than team wOBA—even though wOBA was of course commonly thought to be superior to OPS. His finding was challenged in the comment section of his post, so he ran the comparison again, this time for the 2003-2012 seasons. OPS won again.
The discussion migrated over to Tom Tango’s blog, where it went in a few interesting directions. (Tango is the lead author of The Book). One unresolved question was whether the difference in performance between OPS and wOBA was merely within the margin of error, or in other words, not meaningfully different. Even a finding of equivalence seems meaningful, but if OPS actually fits team run scoring better, that would be even more notable. As far as we can tell, that particular question never got publicly resolved.
Allow us to help. We like the idea of using correlations for statistical comparisons, because correlations are mathematically equivalent to normalized root mean squared error, but are reported on a scale that is easy for the reader to understand. Using a robust Bayesian Pearson correlation, which appears to be even more robust than the Spearman correlation we have been using previously, we took all team batting seasons from 1980-2016, and compared the performance of team OPS versus team wOBA in their respective fits to team runs/PA.
We ran these comparisons in the standard ways that tend to interest us:
- Descriptive Performance: the correlation between the metric and same-year team runs/PA;
- Reliability Performance: the correlation between the metric and itself in the following year; and
- Predictive Performance: the correlation between the metric and the following year’s runs/PA.
Because we coded the analysis in Stan (ok, ok, we used brms), we get the uncertainties for these correlations as a natural byproduct of Bayesian multivariate inference. What do we see when we compare over 1,000 seasons of team OPS/wOBA to team runs/PA? Here are the results:
OPS/wOBA to Team Runs/PA (1980–2016)
Metric | Descriptive | Desc_Err | Reliability | Rel_Err | Predictive | Pred_Err |
OPS | 0.944 | 0.003 | 0.63 | 0.020 | 0.59 | 0.021 |
wOBA | 0.933 | 0.004 | 0.62 | 0.019 | 0.58 | 0.021 |
Morong’s finding was not an anomaly. Put simply, team OPS does better measure team hitting production than team wOBA: the descriptive performance is comfortably outside the margin of error for both statistics, and the reliability and predictive performance measures, while within their respective margins of error, show similar trends.
As noted above, had OPS merely matched wOBA, that would have felt newsworthy, particularly if OPS is as poorly constructed as The Book argues. And yet, the trend over several decades, across time periods of high and low scoring, shows that OPS doesn’t merely hold its own against wOBA: it actually does “better.”
But What Does it Mean for OPS to be “Better”?
At the team level, the conclusion is fairly clear: for measuring raw hitting performance, OPS probably is the better composite metric to use.
If what interests you is individual performance, however, the superiority of OPS becomes less clear.
In the blog thread linked above, Tom Tango, lead author of The Book, contends (in comment no. 32) that OPS has an unfair (and irrelevant) advantage in that it does not count sacrifice flies as plate appearances (because OBP does not count them, and OPS is built on OBP). As such, OPS a) may be tacitly crediting batters for the fortuity of being on a team with teammates who get on base, and b) could therefore overestimate the individual offensive value of a player.
This is a good point, although not an entirely satisfying one. It seems unlikely that sacrifice flies alone could explain the consistent difference in performance. (When we re-ran the comparison above excluding sacrifice flies from OBP/OPS, the results were basically the same). Moreover, if sacrifice flies were the driver of OPS’ (small) advantage in fitting team run scoring, then statistics like wOBA arguably ought to do a better job reflecting the mechanics of sacrifice flies. Sacrifice flies, after all, do not hit themselves. Generating outfield fly balls is a skill, and some batters (usually the better ones) are much better at it than others.
Put another way, fly-ball outs probably are less damaging to a team than ground-ball outs, and that difference, however small, may be worth reflecting, even for individual linear-weights based offensive estimators. Distinguishing ground-ball outs from outfield fly-ball outs is also easy to do, even without stringers or batted-ball data, given the different fielding positions involved.
Perhaps for this reason, Scott Powers’s penalized multinomial estimator distinguishes between ground-ball and fly-ball outs. wOBA, however, declines to so distinguish, perhaps to ensure that all outs equal 0, just like they do for OBP. This is a design choice, and not an unreasonable one, particularly since the authors of The Book are candid about their decision to make it. But it’s not the only choice, and it’s possible that in making this particular choice, wOBA is leaving some accuracy on the table. To the extent OPS incorporates this additional accuracy, however clumsily, that fact is notable and ought to be recognized as such to its credit.
Moving Forward
Our point here is not to force you to choose between OPS, wOBA, or other variants like True Average, since all of them will generally serve you well. Rather, we are trying to lay groundwork for further discussion as to how offensive metrics can be measured, and to remind you of the types of issues we ought to be thinking about as we compare offensive metrics.
Most composite offensive metrics do a good job of measuring hitter quality, at least at the team level, but there are differences that reflect both the quality of their construction and the choices their creators have made. In the coming weeks, we’ll discuss why some of those choices can have astonishing consequences.
Many thanks to the BP Stats Team for peer review and discussion.
Thank you for reading
This is a free article. If you enjoyed it, consider subscribing to Baseball Prospectus. Subscriptions support ongoing public baseball research and analysis in an increasingly proprietary environment.
Subscribe now
Anyways, whether the OBP multiplier is 1.7 or something along those lines, would you be willing to set up a comparison of straight OPS vs mOPS and see if there is any statistical difference there?