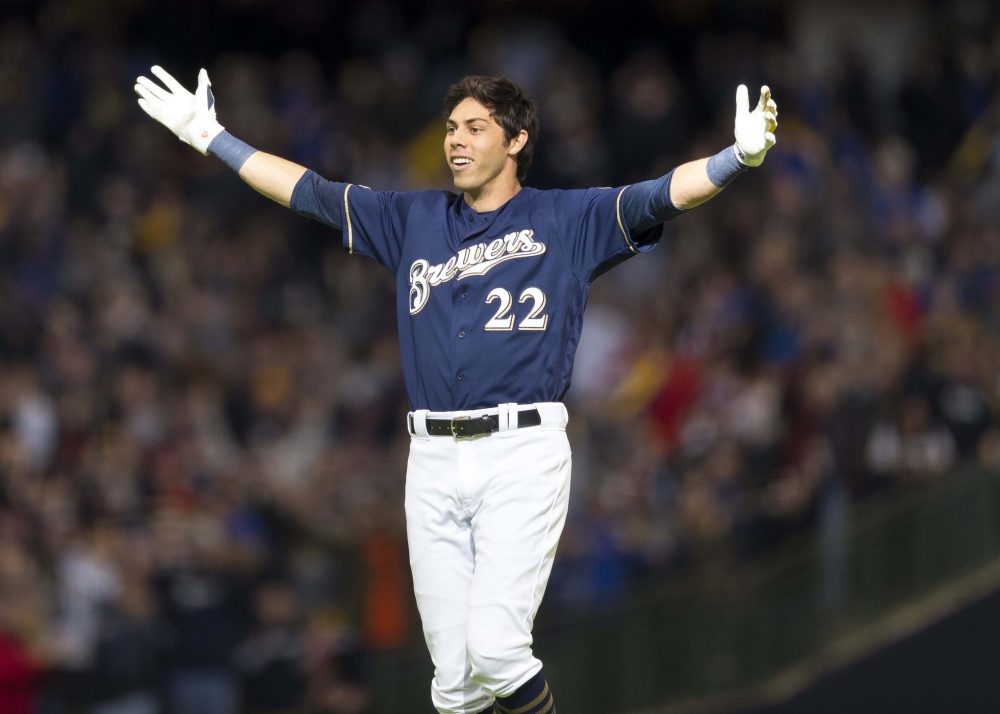
One of the things we like about DRC+, BP’s new flagship batting statistic, is that it reports not only the expected level of contribution for each player, but also how uncertain the DRC system is about that estimate.
Uncertainty quantification is particularly helpful early in the season. In simpler times, readers might choose to “ignore” April statistics, or at least not to take them too seriously. We disagree with the former and somewhat agree with the latter, but rather than sorting statistics into arbitrary calendar bins of “useful” and “not useful,” we prefer to use uncertainty quantification to document how useful they are at any given point in time. This also allows us to watch in real time as a statistic slowly becomes more precise over the course of a season.
There are many ways to represent uncertainty: for now we have chosen to estimate the “standard deviation” around our estimates. The easiest way to think of the standard deviation is that while our estimate reflects the expected DRC+ value, it is substantially more likely than not that the batter’s contribution is inside the range of their DRC+ estimate, plus or minus their DRC_SD, or DRC standard deviation.
Take, for example, Christian Yelich. As reflected in our Sortable Stats, Yelich’s final DRC+ for 2018 was 143, with a standard deviation (DRC_SD) of 10 points. As such, we would say his performance was essentially the same as Jose Ramirez (146 DRC+, DRC_SD of 9), because their respective ranges almost entirely overlap each other: 143 plus or minus 10 points versus 146 plus or minus 9 points.
On the other hand, Yelich almost certainly contributed less than Mike Trout (DRC+ of 183, DRC_SD of 7), whose estimated DRC+, even with a margin of error, is well beyond Yelich’s plus or minus range. For qualified batters, DRC_SD values in the high single digits or low double digits are typical by the end of a recent season.
Not surprisingly, uncertainly is much higher earlier in the season. For example, here are the DRC_SD values for Yelich for each day we have values for so far in 2019:
Calendar Day | Total PAs | DRC+ | DRC_SD |
3/28 | 4 | 353 | 6 |
3/29 | 8 | 341 | 42 |
3/30 | 13 | 218 | 71 |
3/31 | 18 | 250 | 62 |
4/1 | 23 | 214 | 53 |
4/2 | 28 | 219 | 45 |
4/3 | 32 | 214 | 40 |
4/4 | 32 | 212 | 41 |
4/5 | 37 | 197 | 43 |
4/6 | 42 | 156 | 38 |
The early-season uncertainties for DRC+ are high. At first there aren’t enough events to be uncertain about, but once we get above 10 plate appearances or so the system starts to work as expected, shooting up to over 70 points of probable error. Within a week, though, the SD around the DRC+ estimate has worked its way down to the high 30s for a full-time player. That’s remarkably quick progress. This error range continues to decline over the course of the season to the final ranges we discussed above.
It is difficult to distinguish the contribution of two players when their estimates are plus or minus 38 points. Thus, you should indeed be careful about drawing conclusions about players this early in the season. On the other hand, over the coming weeks you can start to feel pretty good about the differences in DRC+ estimates as those SD values continue to decline. And either way, you can at least make your decisions based on actual numbers, rather than arbitrary declarations about “avoiding April statistics” or “waiting until Memorial Day.”
What drives a player’s DRC_SD? There seem to be two primary factors: number of plate appearances and the distribution of batting events for the player. Early on, say in mid-April, everyone has relatively few plate appearances. Thus, DRC variance seems to be driven primarily by what we call “not-in-play” (NIP) events: essentially walk and strikeout rates. By early June, though, the number of plate appearances starts becoming more important, with variance on balls-in-play (BIP) events a somewhat larger factor, and NIP variance becoming less influential. By early August, plate appearances is clearly the most important factor, and becomes the dominant factor by the end of the season.
Keep in mind that all baseball statistics harbor similar or greater uncertainty, at least with respect to their ability to estimate probable player contributions. The difference with DRC+ isn’t that it is uniquely uncertain, but rather that it admits that this uncertainty exists and discloses our best estimate of what that uncertainty is. Other batting statistics tend not to do this. This leaves users to guess at what that uncertainty might be, or even worse, to fall into the trap many readers do and forget that the uncertainty exists at all.
There is plenty more to be said about statistical uncertainty, but we hope this suffices for a primer as the 2019 season continues to unfold.
Thank you for reading
This is a free article. If you enjoyed it, consider subscribing to Baseball Prospectus. Subscriptions support ongoing public baseball research and analysis in an increasingly proprietary environment.
Subscribe now
Thank you!
For example, Pete Alonso is at DRC+ of 186 with SD=40. Should we take that as meaning there's a 5/6 chance he ends up with over 145? And therefore that there's at least a 2/3 chance he's better than Votto (currently at 109/34) this season?
Hi, also, can someone fix this comment entry section? It freaking sucks to have to scroll within the window and everything I press inside the window it jumps to the top of the comments section. It's been like this for at least 5 years and I don't think it's any better on a computer. Reviewing and editing a comment is awful.
I think your question of when DRC+ becomes "useful" for end-of-season expectations is a good one and something I've been thinking about a lot. Pretty good chance you see a comparison among batting stats on that question later this season.
What is "useful" about a stat if not its ability to tell us something about how the player will perform going forward? If all you want is to assess the outcomes, then surely WPA or the raw stats themselves are the appropriate mechanism - if you hit a lot of homers, or added lots of win probability, or held opponents scoreless, then you did well. So inherently, the point of more-complex stats is to look at a sort of projection / counter-factual. That is, to assess how skilled the player is or how well they would do under other potential circumstances.
So to divide it up a little bit, we can ask --
a) what would Pete Alonso's stats have been if he had played the first couple weeks on a different team or in different parks (in this case, DRC+ is a stable projection of value - if you swapped Alonso and Votto you would almost certainly have gotten better results for the Reds and worse ones for the Mets; --
b) what will Pete Alonso's stats look like next week, given how he performed this week? There are two ways to look at this: one is "how stable is DRC+ and DRC+_SD on a weekly / bi-weekly / monthly basis?" and the other is "If DRC+ illuminates some innate skill level, how much does performance at the weekly / bi-weekly / monthly level regress to its prior-year or last-few-years average?"; --
c) how will Pete Alonso's skill evolve over time? This is where the projection system takes over - at some time horizon, there is a fundamental shift in the distribution the DRC is drawn from, and a projection can tell us the arc that shift is likely to take. Still, there's a question here of how helpful early stats are at helping us see which of the possible pre-seasons trajectories this player is taking. In other words, given DRC+ and DRC+_SD so far, how has the probability distribution of PECOTA's percentile outcomes changed?